Palantir partners with data-labeling startup to improve accuracy of AI models
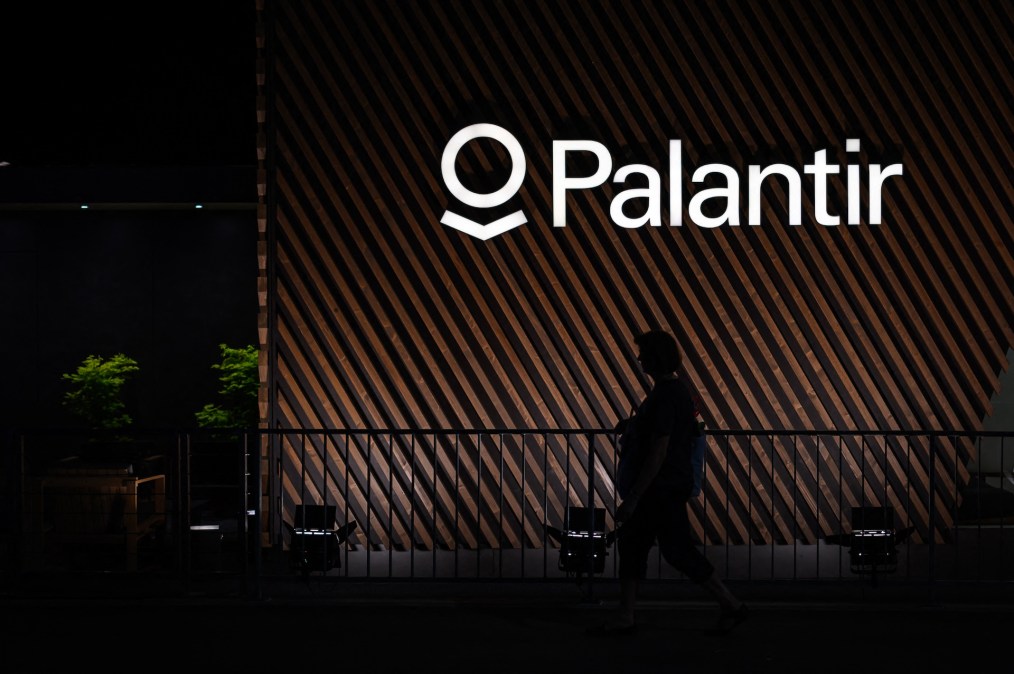
Defense tech company Palantir and startup Enabled Intelligence announced a new partnership aimed at enhancing the quality of data needed to train artificial intelligence models used by organizations within the Defense Department and Intelligence Community.
Under the agreement, federal customers using Palantir’s Foundry system — a software-based data analytics platform that leverages AI and machine learning to automate decision-making — will be able to request data labeling services from Enabled Intelligence. The goal of the partnership is to improve the accuracy of custom AI models built by users by providing them with higher-quality datasets to create and test them with.
“By bringing the Palantir Platform and Enabled Intelligence’s labeling services together in highly secured environments, we believe this will streamline the full cycle of AI model creation and deployment, ensuring that our clients can leverage more precise and actionable insights from their data,” Josh Zavilla, head of Palantir’s national security arm, told DefenseScoop in a statement.
Enabled Intelligence employs a cadre of experts dedicated to annotating multiple data types — including satellite imagery, video, audio, text and more — at a much faster rate than other players in the market, the company’s CEO Peter Kant told DefenseScoop. The impetus for starting Enabled Intelligence came from a gap in the government’s access to accurately labeled data that it needs to train AI models, he said.
“We focus a lot on the quality and the accuracy of the data,” Kant said in an interview. “The better quality of the labeled data, the better and more reliable the AI model is going to be.”
Through the new partnership, government customers are now able to send specific datasets that may need additional labeling directly to Enabled Intelligence’s analysts, Kant explained. Once the data is annotated, the company can push it back to the original users through Foundry so that it can be used to build more accurate artificial intelligence models.
“It’s fully integrated into our labeling pipeline, so we automatically create labeling campaigns to the right people — our employees who know that ontology and know how to do that work with that phenomenology — [and] label it there within Foundry,” Kant said.
The company’s services would be particularly beneficial if a U.S. adversary or rogue actor begins deploying new capabilities that aren’t already included on a training dataset. For example, if American sensors capture imagery indicating that Houthi fighters are using a new small commercial drone as an attack vector, AI models developed for the Maven Smart System or other similar programs might not initially have the right data to support an appropriate response, Kant explained.
While improving the quality of AI has clear advantages for users, Kant emphasized that it can also reduce the overall power needed to run those models. He pointed to the open-source large language model (LLM) developed in China, known as DeepSeek, and claims by its developers that the platform’s performance is comparable to Open AI’s ChatGPT or Google’s Gemini with only a fraction of compute — partly because its developers focused on training data that was well labeled.
“Our customers — especially on the defense and intelligence side — say, ‘Hey, we’re trying to do AI at the edge, or we’re trying to do analysis at the edge.’ You can’t put 1600 GPUs on a [MQ-1 Predator drone], so how do we do this?” Kant said. “One of the ways of doing that has been to really focus on making sure that the data going in is of high quality and can be moved around easily.”
The ability to run AI models with less compute would be particularly beneficial for operators located in remote environments, where it can be difficult to build the necessary infrastructure needed to power them, he added.
“Now we want to use [LLMs] for some real critical systems activities for these missions, and the recognition that the data that goes in and how it’s used to train [AI] and how good it is, it’s been critical — not just in terms of reliability, but also how much compute we need,” Kant said.