The international AI race needs quantum computing
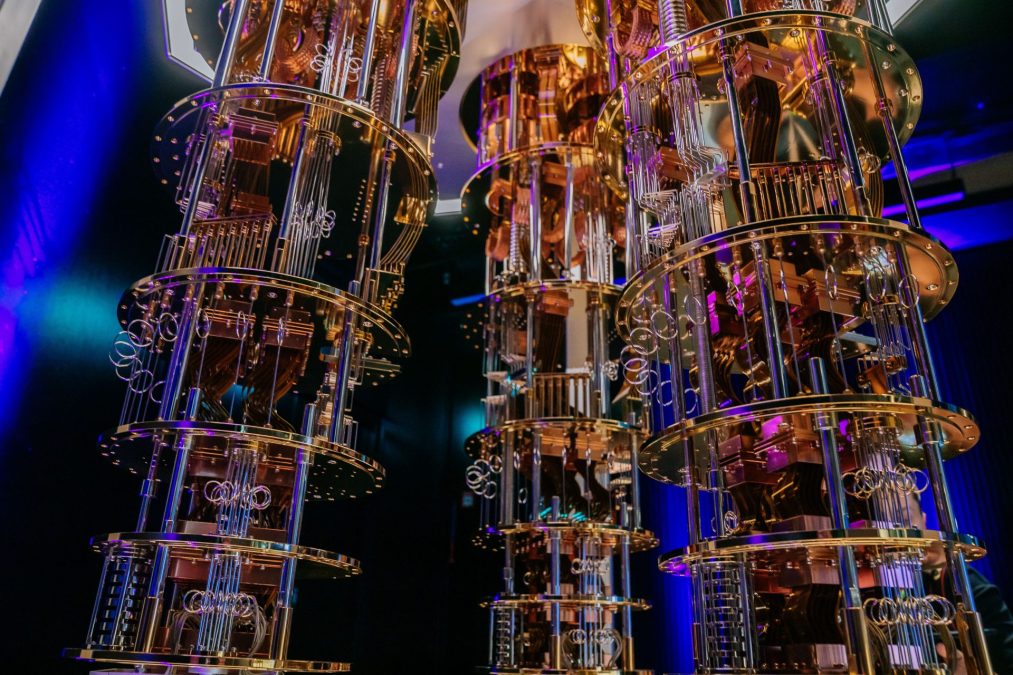
Generative AI technologies have become ubiquitous in our daily lives since OpenAI released ChatGPT two years ago, which now records a staggering 300 million weekly users — roughly equivalent to the population of the U.S.
As our world becomes increasingly powered by generative AI, the most sought-after resource is no longer oil or gold — it’s data. Staying ahead in the AI race requires constant troves of new data to create better generative AI models.
However, we cannot treat AI as just another consumer good designed to make life or work easier, where we simply choose the cheapest or most convenient assistant. The AI technologies we use shape the knowledge we absorb, influence our beliefs, and could become geopolitical tools for misinformation — a national security concern that cannot be overlooked.
For example, the recent high-performing chatbot developed by Chinese company DeepSeek does not provide information about Tiananmen Square and purveys common Chinese Communist Party propaganda about Taiwan and other topics. And yet, days after DeepSeek launched, it became the most popularly downloaded free application in the U.S.
In response, U.S. legislators proposed a bipartisan bill to ban DeepSeek from government devices. The aim is to prevent users from sending heaps of information to DeepSeek and to Chinese state-owned entities. By interacting with DeepSeek over the internet, we are surrendering the single most important resource in maintaining leadership in AI: data.
However, there are three big problems with the data required to train generative AI. First, the world is running out of the high-quality, real-world data required to train models, with Epoch AI predicting we may run out by 2028.
The second problem is that real-world data is inherently flawed and biased because it’s simply a collection of society’s beliefs and actions. Therefore, AI is liable to perpetuate existing political, racist, sexist, and other biases. The current administration has underscored the importance of developing “AI systems that are free from ideological bias or engineered social agendas.”
Third, real-world data is often incomplete. Within the Department of Defense (DOD), intelligence, surveillance and reconnaissance (ISR) systems can face collection gaps, resulting in incomplete data sets (e.g. incomplete satellite image data). In addition, computer security needs — such as identifying network intrusions or malware — may be weakened by incomplete data sets.
The solution to these problems is synthetic data. Synthetic data can augment real-world data by filling in critical gaps, help provide the volume of data needed to train AI and mitigate intrinsic biases. Synthetic data is designed to resemble real-world data and is artificially engineered by computers using algorithms, simulations, or machine learning models. Gartner predicts that more than half of the data used to train AI will be synthetic by 2030.
The benefits of synthetic data generated by today’s “classical” computers are that it’s widely available, affordable, and ideal for small to mid-scale problems with well-structured data. The drawbacks are that classical synthetic data can be less complex and diverse than real-world data, can struggle to capture the high-dimensional patterns needed for training AI, and may face future challenges in scaling quickly to meet growing data demands.
Quantum computing is the solution to these challenges. Quantum computers will generate higher volumes of data and higher quality data than classical synthetic data.
“The future of generative AI training lies in combining real-world data with both classical and quantum synthetic data,” says Dr. Graham Enos, vice president of quantum solutions at Strangeworks and a former DOD mathematician. “As quantum computing advances, quantum synthetic data will increasingly dominate the synthetic data used to train AI. What’s exciting is that synthetic data generation is one of the most immediate and practical applications of quantum computers.”
The seemingly otherworldly properties of quantum computers make them ideal for the machine learning and simulation tasks that generate synthetic data. Unlike classical computers, which rely on bits that are either 0 or 1, quantum computers use qubits that can exist in a superposition of both states simultaneously, providing exponentially greater computing power. Entanglement is another critical property of quantum computing that allows qubits to represent more complex data distributions, enabling more complicated calculations than classical computers. By leveraging both superposition and entanglement, quantum computers can double their compute power simply by adding one qubit — in contrast, classical systems require doubling the number of transistors to double compute power.
Five years ago, the largest quantum computer was Google’s 53-qubit Sycamore chip that demonstrated “beyond classical” performance on a computational benchmark. The largest machines built today, from IBM and Atom Computing, boast upwards of 1,000 qubits. While quantum computers are not yet outperforming classical computers for practical applications, including generating meaningful quantum synthetic data for commercial AI training, they are quickly approaching that moment.
Recently, quantum computing company Quantinuum, a spinoff from Honeywell, announced that data from its H2 quantum computer can train AI systems using its Generative Quantum AI framework.
In the announcement, Dr. Thomas Ehmer from the healthcare business sector of Merck KGaA, Darmstadt is quoted as saying, “While some may suggest that a standalone quantum computer is still years away, the commercial opportunities from this breakthrough are here and now…the [Quantinuum] Helios system, launching later this year will hopefully enable AI to be used in unprecedented ways and unlocking transformative potential across industries.”
Similarly, in work partially funded by the U.S. government, Rigetti (RGTI) used a quantum neural network to generate synthetic data and fill gaps in global weather radar coverage, matching the performance of a classical baseline model. (These quantum machine learning methods from Rigetti are available on Strangeworks.)
This type of work is directly applicable to enhancing C4ISR capabilities by leveraging advanced computer vision techniques to analyze complex sensor data. For example, programs like Project Maven use synthetic data to train AI models that interpret full-motion video (FMV), synthetic aperture radar (SAR) imagery, and other intelligence sources.
The impacts of quantum computing go beyond improving AI models. Additional defense-related applications include cybersecurity threat detection, adversarial intent prediction, cryptanalysis, electromagnetic spectrum operations, and many more.
Yet, China is outspending the U.S. four to one in federal quantum technology investment and is steadily closing the technology gap. If the U.S. wants to continue to lead the quantum computing race and be the first to fully leverage the power of quantum synthetic data, we must bolster public and private investment in quantum technologies.
First, due to the time and effort needed to develop quantum workflows, government and private organizations should start working with experts today to identify use cases and build the hybrid infrastructure needed for rapid adoption of quantum data.
Second, the U.S. must reauthorize the National Quantum Initiative (NQI) Act, which accounts for about half of federal investment in quantum technologies. The initial NQI Act, which expired in 2023, was signed into law by President Donald Trump in 2018 and resulted in meaningful progress.
Lastly, Congress should increase funding to both DOD and the Department of Energy by passing the newly introduced bipartisan DOE Quantum Leadership Act as well as the Defense Quantum Acceleration Act. The latter was introduced by Rep. Elise Stefanik and Sen. Marsha Blackburn in April, and the legislation is intended to “supercharge the Department of Defense’s approach to quantum technology and advance U.S. national security.”
Without a recommitment to federal investment in quantum technologies, the U.S. risks another DeepSeek moment with both quantum and AI.